Introduction
The  correlation coefficient is a
generalization of Pearson's correlation coefficient, see for example http://mathworld.wolfram.com/CorrelationCoefficient.html
and have similar properties in respect to least squares as in the Pearson case,
see eg. http://mathworld.wolfram.com/LeastSquaresFitting.html.
Here the correlation coefficient extends to K-dimensional
data points and an expected covariance can be defined:
where

and elements of the NxK table
 .
with columns
 . The correlation coefficient is
For a linear fit
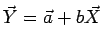
it can be shown that it has similar properties as in the Pearson case, where

can be discretized to obtain an equivalent for Matthews correlation coefficient:
where

are the elements of the confusion matrix.
For more details see the reference below:
Reference
Comparing
two K-category assignments by a K-category correlation coefficient
J. Gorodkin, Computational Biology and Chemistry, 28:367-374, 2004.
Abstract of the reference:
Predicted assignments of biological sequences are often evaluated by Matthews
correlation coefficient. However, Matthews correlation coefficient applies only
to cases where the assignments belong to two categories, and cases with more
than two categories are often artificially forced into two categories by
considering what belongs and what does not belong to one of the categories,
leading to the loss of information. Here, an extended correlation coefficient
that applies to K-categories is proposed, and this measure is shown to be
highly applicable for evaluating prediction of RNA secondary structure in cases
where some predicted pairs go into the category ``unknown'' due to lack of
reliability in predicted pairs or unpaired residues. Hence, predicting base
pairs of RNA secondary structure can be a three category problem. The measure
is further shown to be well in agreement with existing performance measures
used for ranking protein secondary structure predictions.
Comments, questions, etc., email
webmaster@rk.kvl.dk.
|